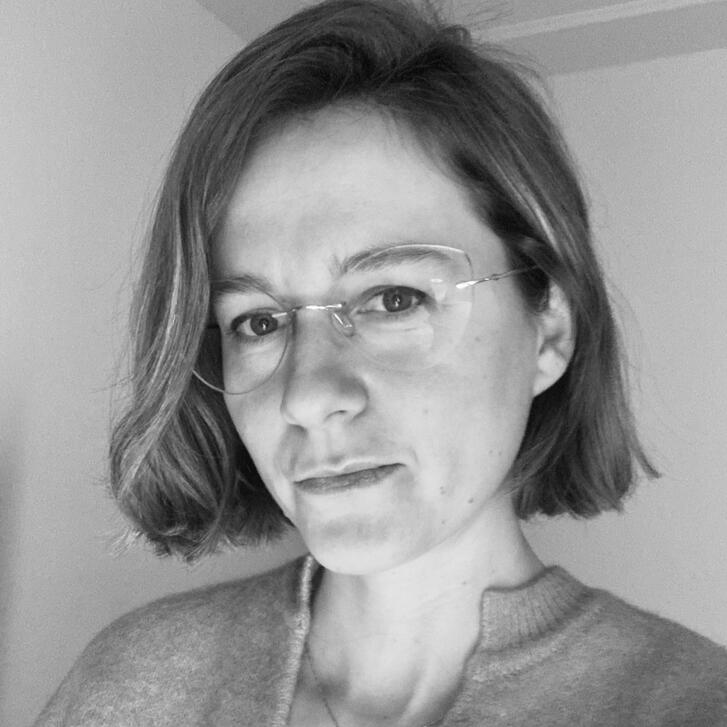
Bojana Grujičić
I am in the last stages of my PhD in philosophy of neuroscience within the framework of Max Planck School of Cognition, conducting my research at Humboldt-Universität zu Berlin and University College London.I am also a researcher at the Excellence Cluster “Science of Intelligence” at Technische Universität Berlin.I am interested in the use of deep learning for neuroscientific goals, explanation in neuroscience, model-based neuroscientific reasoning, representational alignment of neural networks and brains and compositionality-related issues.
Synthese
Deep convolutional neural networks are not mechanistic explanations of object recognition
Given the extent of using deep convolutional neural networks to model the mechanism of object recognition, it becomes important to analyse the evidence of their similarity and the explanatory potential of these models. I focus on one frequent method of their comparison – representational similarity analysis, and I argue, first, that it underdetermines these models as how-actually mechanistic explanations. This happens because different similarity measures in this framework pick out different mechanisms across DCNNs and the brain in order to correspond them, and there is no arbitration between them in terms of relevance for object recognition. Second, the reason similarity measures are underdetermining to a large degree stems from the highly idealised nature of these models, which undermines their status as how-possibly mechanistic explanatory models of object recognition as well. Thus, building models with more theoretical consideration and choosing relevant similarity measures may bring us closer to the goal of reaching a mechanistic explanation.
Forthcoming in The Routledge Handbook of Causality and Causal Methods (Eds. Illari, P & Russo, F)
Using deep neural networks and similarity metrics to predict and control brain responses
With Phyllis IllariIn the last ten years there has been an increase in using artificial neural networks to model brain mechanisms, giving rise to a deep learning revolution in neuroscience. This chapter focuses on the ways convolutional deep neural networks (DCNNs) have been used in visual neuroscience. A particular challenge in this developing field is the measurement of similarity between DCNNs and the brain. We survey similarity measures neuroscientists use, and analyse their merit for the goals of causal explanation, prediction and control. In particular, we focus on two recent intervention-based methods of comparing DCNNs and the brain that are based on linear mapping (Bashivan et al., 2019, Sexton and Love, 2022), and analyse whether this is an improvement. While we conclude explanation has not been reached for reasons of underdetermination, progress has been made with regards to prediction and control.
Frontiers in Psychology
Clarifying the nature of stochastic fluctuations and accumulation processes in spontaneous movements
With Carsten Bogler & John-Dylan HaynesExperiments on choice-predictive brain signals have played an important role in the debate on free will. In a seminal study, Benjamin Libet and colleagues found that a negative-going EEG signal, the readiness potential (RP), can be observed over motor-related brain regions even hundreds of ms before the time of the conscious decision to move. If the early onset of the readiness potential is taken as an indicator of the “brain’s decision to move” this could mean that this decision is made early, by unconscious brain activity, rather than later, at the time when the subject believes to have decided. However, an alternative kind of interpretation, involving ongoing stochastic fluctuations, has recently been brought to light. This stochastic decision model (SDM) takes its inspiration from leaky accumulator models of perceptual decision making. It suggests that the RP originates from an accumulation of ongoing stochastic fluctuations. In this view, the decision happens only at a much later stage when an accumulated noisy signal (plus imperative) reaches a threshold. Here, we clarify a number of confusions regarding both the evidence for the stochastic decision model as well as the interpretation that it offers. We will explore several points that we feel are in need of clarification: (a) the empirical evidence for the role of stochastic fluctuations is so far only indirect; (b) the interpretation of animal studies is unclear; (c) a model that is deterministic during the accumulation stage can explain the data in a similar way; (d) the primary focus in the literature has been on the role of random fluctuations whereas the deterministic aspects of the model have been largely ignored; (e) contrary to the original interpretation, the deterministic component of the model is quantitatively the dominant input into the accumulator; and finally (f) there is confusion regarding the role of “imperative” in the SDM and its link to “evidence” in perceptual decision making. Our aim is not to rehabilitate the role of the RP in the free will debate. Rather we aim to address some confusions regarding the evidence for accumulators playing a role in these preparatory brain processes.
Work in progress
Deep learning and scientific understandingAn account of surrogative reasoning with deep neural networksAn account of accurate representation with deep neural networks